Graph-based quantification of surface cracks in concrete structures
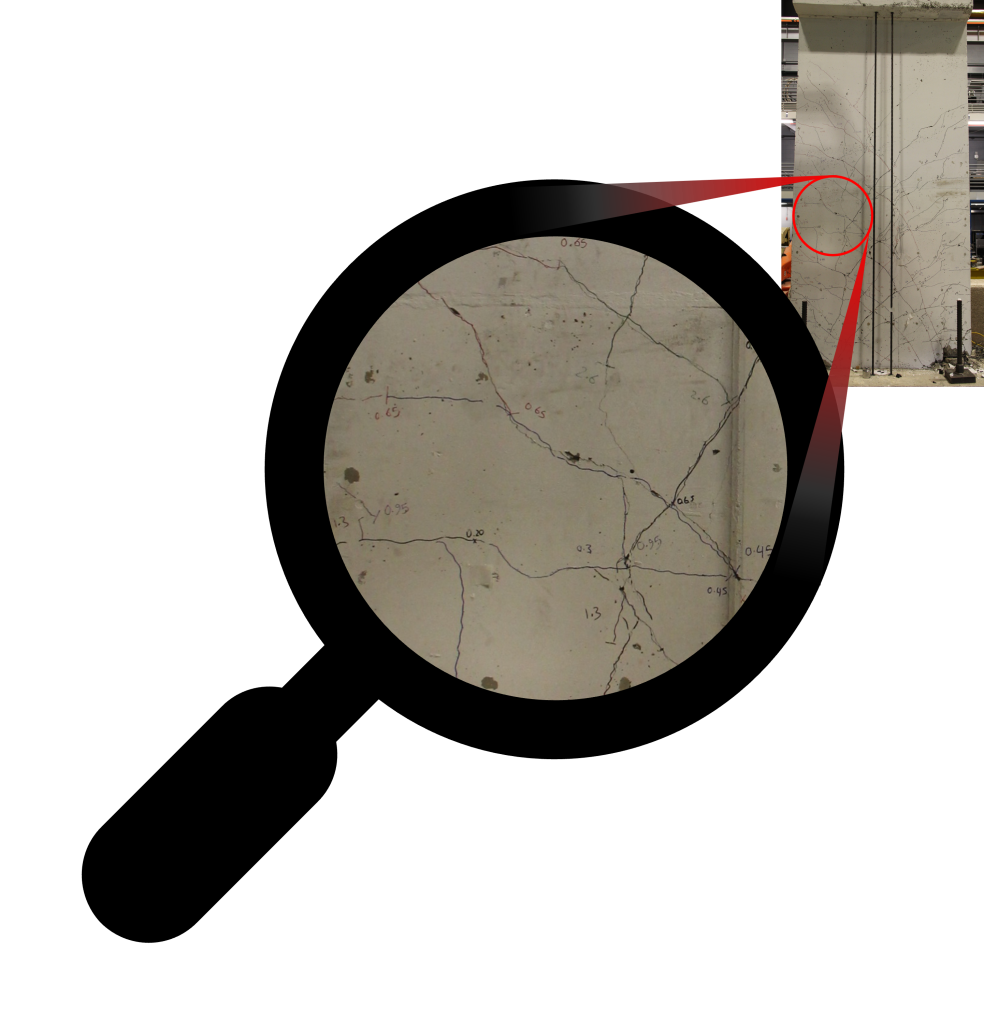
This project develops a novel method for vision-based nondestructive evaluation of concrete structures. As infrastructures are aging and threats (e.g., hurricanes) are intensifying (e.g., due to climate change), the importance of enhancing the speed and reliability of monitoring and maintenance procedures is increasing. The subjective and manual aspects of current structural assessment methods compromise the reliability of these practices. Therefore, developing objective and autonomous methods that facilitate robust and reliable infrastructure assessment is a necessity. The inevitable fact is that concrete structures crack due to different reasons, and currently, there is no structural model for cracked concrete that takes crack patterns as input. To this end, this research proposes a physics-guided artificial intelligence (AI) that uses crack patterns to predict the parameters of an element-level structural model. The model can then be integrated with existing numerical modeling tools and existing performance-based design procedures for assessment and/or retrofit.
Robotic-based monitoring of infrastructures
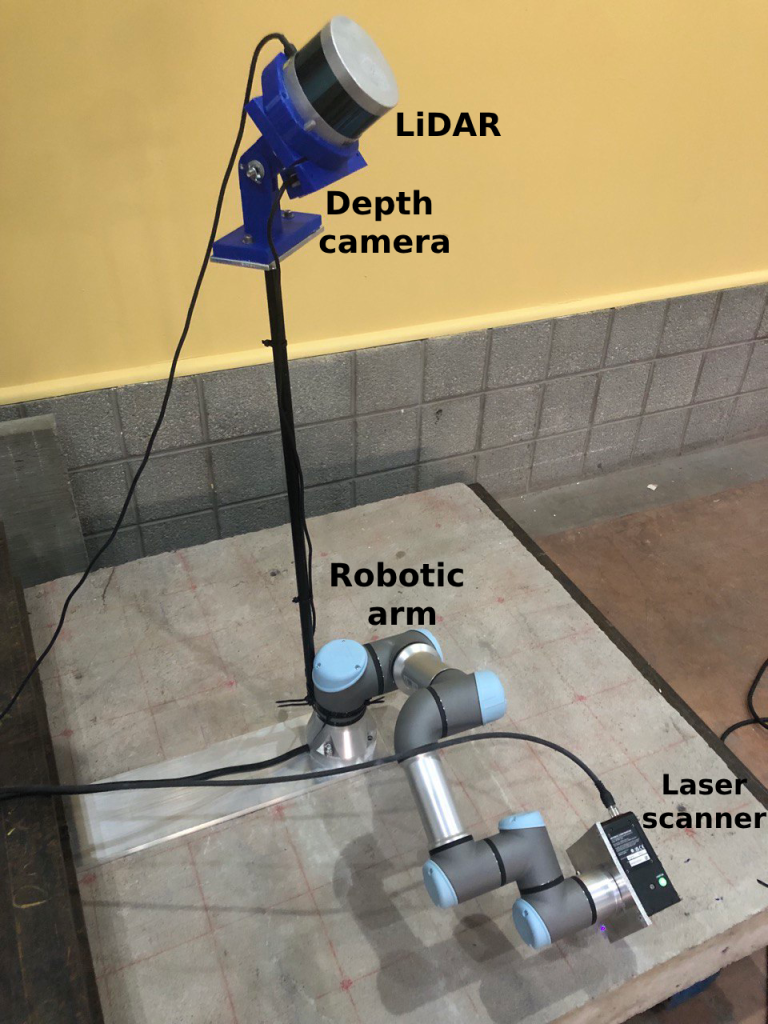
This research focuses on bridging the scale difference in data collection from small defects of large civil infrastructures. The idea is to use the domain knowledge and artificial intelligence (AI) to identify indications of defects in low resolution scans and prioritizes high-resolution robotic scans accordingly. As a showcase, this project introduces a multi-scale robotic approach for measuring the width, length, shape, and profile of hairline cracks in concrete structures.