The use of drones also known as Unmanned Aerial Vehicles (UAVs) in industries has highlighted the need, for detection systems to ensure their safe interaction with manned aircraft and their operation in sensitive or populated areas. Developing systems heavily depends on having a range of realistic datasets that can effectively train and assess drone detection algorithms. Despite advancements in drone detection research there is still a lack of datasets that accurately reflect the challenges faced in real world situations in long range detection under varying weather conditions.
The Long Range Drone Detection (LRDD) V2 dataset, which includes 39,516 annotated images, represents a significant enhancement in drone detection research. It is uniquely characterized by the inclusion of range information for over 8,000 images—a feature that is rarely found in existing drone datasets. Designed to tackle the intricacies of long-range drone identification, the LRDD V2 dataset is comprised of a diverse collection of images that address a multitude of real-world challenges. These challenges include long-distance captures essential for developing distant UAV detection algorithms, moving camera dynamics to mirror real-world operational surveillance, and scenarios featuring occlusion, background blending, diverse illumination, and variable weather conditions. The dataset also presents the complex task of multiple object detection and includes images from a variety of environments. The incorporation of explicit range information significantly enriches the dataset, offering a unique and valuable resource for the research community to develop, train, and benchmark sophisticated algorithms for UAV surveillance and security applications in realistic settings. This dataset not only extends the capabilities of current UAV detection methods but also provides an extensive platform for advancing drone detection technologies within the field.
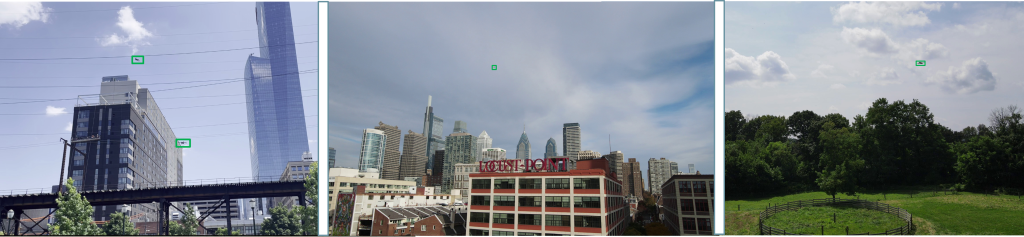
In order to download the dataset, please fill out the form below:
https://forms.gle/fBpBWap3S2Vuy9ou7
After you submit this form, we will email you the link to access the dataset.
Should you encounter any issues, feel free to reach out by sending an email to ar3755@drexel.edu.