Solid-state inorganic compounds are crucial to the design of functional devices to meet societal needs for clean energy, environmental sustainability, and human welfare. The discovery and efficient synthesis of novel inorganic compounds often catalyzes breakthroughs in material innovation. With the rapid progress in high-throughput density functional theory (DFT) calculations and the correspondingly emerged databases, new compound chemistries and phases with exceptional functional properties are being identified and designed at an unprecedented pace. Nevertheless, these computational findings have rarely been converted into practical material applications by missing key knowledge regarding material synthesizability. Currently, there are no generally applicable frameworks to efficiently predict synthesis pathways for solid-state inorganic compounds, particularly for those with phase stability only in a certain window of synthesis conditions. This significantly bottlenecks the fulfillment of the extensive potential of computational materials design in the advancement of novel, functional solid-state materials. The current focus is on demonstrating this framework through the successful synthesis of a group of transition metal oxynitrides, which are computationally designed and highly interesting for energy conversion applications but have not yet been fully realized experimentally, as well as two-dimensional(2D) transition metal carbides and nitrides (MXenes). This research is a team effort in collaboration with Prof. Jill Wenderott, Prof. Yury Gogotsi, and Prof. Kaidi Xu at Drexel University.
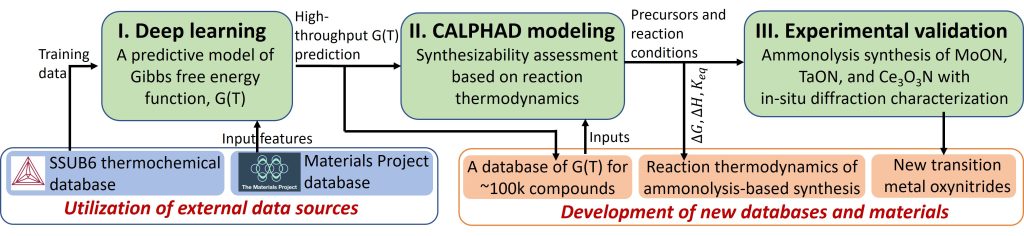
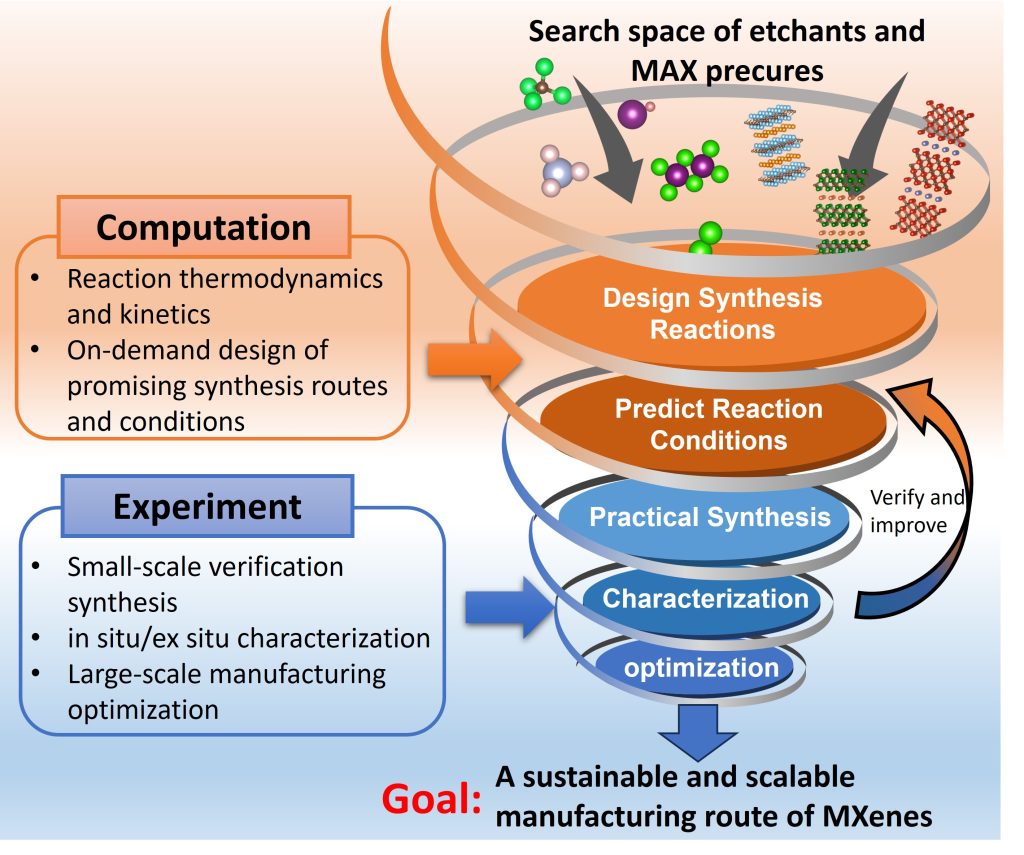